In recent years, Artificial Intelligence (AI) has emerged as a game-changer in various industries, and healthcare is no exception. With its potential to revolutionize patient care, AI is poised to transform the way healthcare is delivered, improve patient outcomes, and empower healthcare providers. In this article, we will explore seven crucial areas where AI can have a profound impact on healthcare and how its implementation can change people’s perception of AI in healthcare.
Data Analysis and Insights:
The vast amount of healthcare data generated daily presents both challenges and opportunities. AI can analyze this data to uncover hidden patterns, identify trends, and derive actionable insights. By leveraging advanced algorithms and machine learning techniques, AI can assist healthcare professionals in making more informed decisions, leading to improved patient care and outcomes. Implementation involves integrating AI systems into existing healthcare infrastructure, enabling seamless data analysis and offering intuitive visualizations and reports to aid decision-making.
Clinical Decision Support:
In the complex world of healthcare, AI can provide valuable decision support to healthcare providers at the point of care. By integrating with electronic health records (EHRs) and leveraging patient-specific data, AI algorithms can suggest evidence-based treatment options, alert clinicians to potential drug interactions or allergies, and provide real-time access to relevant clinical guidelines. The implementation requires developing AI models that can quickly process patient data and provide accurate and relevant recommendations, along with user-friendly interfaces that seamlessly integrate into existing clinical workflows.
Disease Detection and Diagnosis:
Early detection and accurate diagnosis are critical in effectively treating diseases. AI algorithms can analyze medical images, such as X-rays, CT scans, or MRIs, with a level of precision and speed that surpasses human capabilities. By aiding healthcare professionals in identifying abnormalities and potential indicators of diseases like cancer, cardiovascular conditions, or neurological disorders, AI can significantly improve diagnosis accuracy and treatment outcomes. Implementation involves training AI models on large datasets of medical images, integrating them into existing radiology and diagnostic workflows, and validating their performance in clinical settings.
Remote Patient Monitoring:
The rise of telehealth and remote care has opened up new possibilities for providing healthcare beyond traditional clinical settings. AI can play a pivotal role in remote patient monitoring by analyzing data from wearable devices, sensors, and other remote monitoring technologies. By continuously monitoring vital signs, sleep patterns, glucose levels, and more, AI can detect deviations from normal ranges and alert healthcare providers in real-time, facilitating early interventions and proactive care. Implementation requires integrating AI systems with remote monitoring devices, ensuring data security and privacy, and providing seamless data transmission and analysis capabilities.
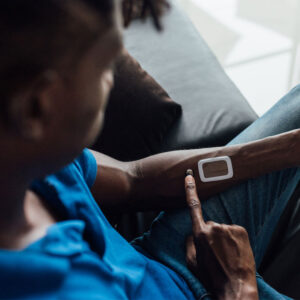
Drug Discovery and Development:
The process of drug discovery and development is a complex and time-consuming endeavor. AI can accelerate this process by analyzing vast amounts of molecular structures, genetic information, and clinical trial data. By leveraging AI techniques like machine learning, AI can assist in predicting drug efficacy, identifying potential side effects, and optimizing treatment protocols. Implementation involves collaborating with pharmaceutical companies, academic institutions, and regulatory bodies to build comprehensive datasets, develop AI models, and validate their findings in preclinical and clinical settings.
Healthcare Resource Allocation:
Efficient resource allocation is a significant challenge in healthcare. AI can analyze historical data, patient demographics, and treatment outcomes to provide insights that inform resource allocation decisions. By optimizing the allocation of healthcare resources, such as hospital beds, staff, and equipment, AI can enhance efficiency, reduce waiting times, and improve patient satisfaction. Implementation involves integrating AI systems with existing healthcare information systems, ensuring data quality and accuracy, and providing decision support tools for healthcare administrators and policymakers.
Patient Education and Support:
Empowering patients with knowledge and personalized support is crucial for their active participation in healthcare. AI can leverage patient data to provide tailored health information, education, and self-management strategies. By offering personalized recommendations, lifestyle advice, and disease management tools, AI can support patients in making informed decisions about their health and well-being. Implementation requires designing user-friendly AI interfaces, ensuring privacy and security of patient data, and collaborating with healthcare providers to incorporate AI-driven patient education and support systems into their practice.
Artificial Intelligence in healthcare is not just one system. It’s a combination of them that serves a specific purpose. The three main AI systems with high importance in healthcare are machine learning, natural language processing (NLP), and rule based expert systems.
Different Types of AI in Modern Healthcare
Machine Learning:
Machine learning is a branch of AI that involves the development of algorithms capable of learning from data and making predictions or decisions without explicit programming. It’s a broad technique which forms a fundamental part of various AI approaches.
It is usually categorized into three different types of machine learning algorithms used in artificial intelligence:
- Supervised Learning: In this approach, the AI system learns from labeled data with known outcomes, allowing it to make predictions or classifications on new, unseen data. Supervised learning algorithms can be used for tasks like disease classification based on medical images or predicting patient outcomes based on electronic health records.
- Unsupervised Learning: Unsupervised learning algorithms are used when the data is unlabeled or when the AI system needs to discover hidden patterns and relationships within the data. These algorithms can aid in clustering patient data for personalized treatment approaches for identifying anomalies in large datasets for disease surveillance.
- Reinforcement Learning: Reinforcement learning involves an AI system learning through interactions with an environment, receiving feedback or rewards based on its actions. In healthcare, reinforcement learning can be employed to optimize treatment strategies or personalized interventions based on continuous feedback from patient responses.
Natural Language Processing (NLP):
NLP focuses on enabling computers to understand and process human language in both written and spoken forms. In healthcare, NLP can extract information from clinical notes, research papers, or patient-generated data. It has enhanced patient engagement through sentiment analysis, enabling healthcare providers to address patient concerns effectively. As well as efficient analysis of vast amounts of textual data, helping healthcare professionals stay updated on the latest research and clinical guidelines. Complexity of medical language and diverse healthcare terminologies pose challenges for accurate interpretation and extraction of information.
NLP can be used in various ways including:
- Text Classification: NLP algorithms can categorize and classify medical literature, patient records, or social media data, assisting in information retrieval and summarization.
- Named Entity Recognition (NER): NER algorithms identify and extract specific entities, such as disease names, medication names, or patient demographics, from unstructured text, enabling better organization and analysis of healthcare information.
- Sentiment Analysis: Sentiment analysis algorithms can gauge the emotional tone of patient reviews, social media posts, or physician notes, providing insights into patient experiences and satisfaction levels.
Rule-based Expert Systems:
Rule-based expert systems rely on a set of predefined rules and logical inferences to make decisions or provide recommendations. These systems incorporate human expertise and domain-specific knowledge. It has reliable decision-making, especially in situations where clear rules and guidelines exist. However limited ability to handle complex and uncertain scenarios that may not fit predefined rules, and the manual rule development can be time consuming are drawbacks.
Here are two different types of rule-based expert systems:
- Knowledge Base: Rule-based expert systems include a knowledge base containing a collection of rules and facts relevant to the healthcare domain.
- Inference Engine: The inference engine processes the input and applies the rules to draw conclusions or make decisions based on the available information.
Implementing AI in healthcare brings forth several challenges that need to be addressed. One significant challenge is ensuring the quality and availability of data, as healthcare data can be incomplete, inconsistent, or fragmented across different systems. Regulatory and ethical considerations are paramount to protect patient privacy, ensure data security, and promote fair usage of AI algorithms. Another challenge lies in the interoperability and integration of AI systems with existing healthcare infrastructure, such as electronic health records and clinical workflows. Maintaining system stability while achieving seamless integration is crucial. Moreover, building trust and acceptance among healthcare professionals is essential for successful implementation. Collaboration between AI systems and human experts is necessary to ensure that AI-driven recommendations are understood, verified, and appropriately integrated into clinical decision-making processes. By addressing these challenges, we can unlock the transformative potential of AI in healthcare, revolutionizing patient care, improving outcomes, and driving advancements in the field.
AI in healthcare brings forth various implications, encompassing ethical, medical, and occupational considerations. These implications can shape the perception and acceptance of AI technologies in healthcare.
Ethical Implications:
- Privacy and Data Security: The use of AI in healthcare involves handling sensitive patient data, raising concerns about privacy breaches or unauthorized access. Striking the right balance between utilizing data for improving patient care and ensuring stringent data security measures is crucial.
- Bias and Fairness: AI systems can inadvertently amplify existing biases in healthcare data, leading to disparities in diagnosis, treatment, or access to care. It is vital to mitigate bias and ensure fairness in AI algorithms to promote equitable healthcare practices.
- Informed Consent and Transparency: As AI systems impact patient care, ensuring that patients are informed about the involvement of AI in their healthcare decisions becomes essential. Transparency in how AI systems reach their conclusions or recommendations is crucial to build trust and maintain patient autonomy.
Medical Implications:
- Diagnostic Accuracy and Reliability: While AI can aid in diagnostics, it is essential to establish the accuracy and reliability of AI systems. Validating AI algorithms against gold-standard diagnostic methods is crucial to ensure patient safety and prevent misdiagnosis or improper treatment.
- Clinical Decision-Making: The integration of AI systems in clinical decision-making raises questions about the appropriate balance between AI-driven recommendations and the expertise of healthcare professionals. The collaboration between AI systems and human experts is crucial to ensure optimal patient outcomes.
- Continuity of Care: AI systems should be designed to seamlessly integrate with existing healthcare workflows and systems to maintain the continuity of care. Ensuring that AI complements the existing healthcare infrastructure rather than disrupting it is vital.
Occupational Implications:
- Workforce Impact: The introduction of AI technologies may create concerns about job displacement among healthcare professionals. However, AI can augment healthcare professionals’ capabilities by handling repetitive tasks, allowing them to focus on more complex and critical aspects of patient care.
- Skill Development and Training: The integration of AI necessitates upskilling and retraining healthcare professionals to effectively work alongside AI systems. Providing adequate training and resources to healthcare professionals can empower them to adapt to AI-driven healthcare environments.
- Liability and Accountability: The use of AI in healthcare raises questions about liability and accountability in case of adverse events or errors. Determining responsibility in situations involving AI-driven decisions requires clear policies and guidelines.
Moving Forward with Ethical AI in Healthcare
Addressing these ethical, medical, and occupational implications is crucial for the successful implementation of AI in healthcare. It necessitates collaboration between healthcare professionals, policymakers, and technology developers to ensure that AI technologies are deployed in an ethically sound and patient-centric manner, while also respecting the expertise and values of healthcare professionals. By navigating these implications thoughtfully, AI can enhance healthcare delivery, improve patient outcomes, and promote a harmonious coexistence between technology and human expertise.
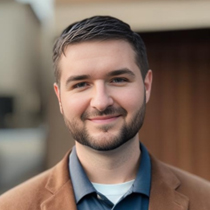
With over 16 years in the industry, Jameson Lee has honed his skills in IT management, project execution, and strategic planning. His ability to align technology initiatives with business goals has consistently delivered remarkable results for organizations across various sectors.
Jameson’s educational background includes an Associate of Applied Science degree in Computer Networking Systems, providing him with a solid foundation in technical concepts and best practices. Complementing his technical acumen, he has also completed coursework in Business Administration, equipping him with a well-rounded understanding of the operational aspects of running successful businesses.
Driven by a commitment to staying ahead of industry trends, Jameson actively pursues professional certifications and continuous learning opportunities. His credentials include CompTIA A+, N+, and Security+, along with MCP and MCTS certifications. This dedication ensures that he remains at the forefront of technological advancements, enabling him to offer innovative solutions to complex challenges.
What sets Jameson apart is his personable approach to working with clients. He believes in fostering strong relationships and effective communication, collaborating closely with stakeholders to understand their unique needs, and provide tailored technology solutions. By building trust and understanding, Jameson ensures that every project is aligned with the client’s vision and objectives.
Throughout his career, Jameson has successfully led teams and implemented robust frameworks to optimize performance and achieve remarkable technological initiatives. Whether it’s streamlining operations, enhancing cybersecurity measures, or implementing cutting-edge software solutions, Jameson has consistently delivered tangible outcomes for his clients.
As a trusted IT partner, Jameson’s mission is to empower businesses with technology solutions that drive growth, efficiency, and competitive advantage. With his expertise, dedication, and personable approach, Jameson Lee is the catalyst for transforming your business through the power of technology.